Elements Of Statistical Computing Pdf Printer
9/14/2018 admin
Elements Of Statistical Computing Pdf Printer 7,1/10 8389reviews
Survey of statistical concepts, data analysis, designed and observational studies and statistical models. Statistical computing using a statistical package such as R or a spreadsheet. Topics to be covered include data summary and visualization, study design, elementary probability, categorical data, comparative experiments, multiple linear regression, analysis of variance, statistical inferences and model diagnostics. Hpi Rc Cars Dvd Version 3. May be taken as a first statistics course for quantitatively oriented students, or as a second course to follow a basic concepts course. Credit is not given for both and.
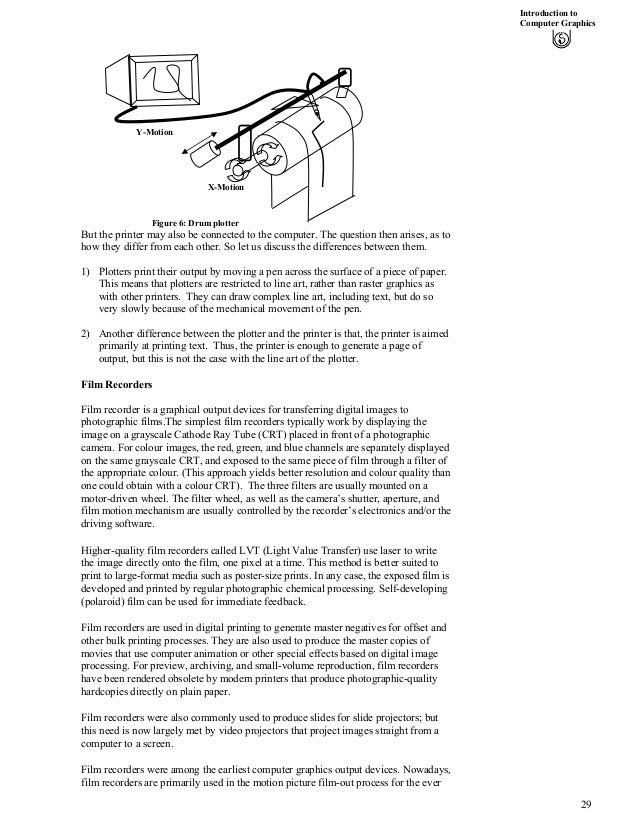
Comparison of Statistical Models for Writer Veri. Elements, or features, that. Computing For Psychologists Statistical Analysis Using Spss And Minitab Download Computing For Psychologists Statistical Analysis Using Spss And Minitab in pdf, reading online Computing For Psychologists.
This course satisfies the General Education Criteria for: Quantitative Reasoning I. Statisticians must be savvy in programming methods useful to the wide variety of analysis that they will be expected to perform. This course provides the foundation for writing and packaging statistical algorithms through the creation of functions and object oriented programming. Fundamental programming techniques and considerations will be emphasized.
Students will also create dynamic reports that encapsulate their implemented algorithms. Zend Framework Encrypt Decrypt Php here. Students must have access to a computer on which they can install software. Systematic, calculus-based coverage of the more widely used methods of applied statistics, including simple and multiple regression, correlation, analysis of variance and covariance, multiple comparisons, goodness of fit tests, contingency tables, nonparametric procedures, and power of tests; emphasizes when and why various tests are appropriate and how they are used. 3 undergraduate hours. 4 graduate hours.
Prerequisite: or; or equivalent; knowledge of basic matrix manipulations; or consent of instructor. Introduction to the concepts and methodology of Bayesian statistics, for students with fundamental knowledge of mathematical statistics. Topics include Bayes' rule, prior and posterior distributions, conjugacy, Bayesian point estimates and intervals, Bayesian hypothesis testing, noninformative priors, practical Markov chain Monte Carlo, hierarchical models and model graphs, and more advanced topics as time permits. Implementations in R and specialized simulation software. 3 undergraduate hours. 4 graduate hours.
Prerequisite: and knowledge of R. Topics in supervised and unsupervised learning are covered, including logistic regression, support vector machines, classification trees and nonparametric regression. Model building and feature selection are discussed for these techniques, with a focus on regularization methods, such as lasso and ridge regression, as well as methods for model selection and assessment using cross validation. Cluster analysis and principal components analysis are introduced as examples of unsupervised learning.
3 undergraduate hours. 4 graduate hours. Prerequisite:, and either. A stochastic process is a random process that represents the evolution of some system over time. Topics may include discrete-time and continuous-time Markov chains, birth-and-death chains, branching chains, stationary distributions, random walks, Markov pure jump processes, birth-and-death processes, renewal processes, Poisson process, queues, second order processes, Brownian motion (Wiener process), and Ito's lemma. 3 undergraduate hours.
4 graduate hours. Prerequisite: required, preferred, and (or equivalent knowledge of Linear Algebra) highly recommended. The critical elements of data storage, data cleaning, and data extractions that ultimately lead to data analysis are presented.
Includes basic theory and methods of databases, auditing and querying databases, as well as data management and data preparation using standard large-scale statistical software. Students will gain competency in the skills required in storing, cleaning, and managing data, all of which are required prior to data analysis. 3 undergraduate hours. 4 graduate hours. Examines the methods of data management and analysis for 'big data', characterized by high volume, variety, velocity, and veracity. Attention will be focused on advanced statistical analysis and visualization in data science applications employing parallel processing, storage and distribution techniques necessary for analysis of massive data sets. Data mining techniques, machine learning methods, and streaming technologies will be utilized for real-time analysis.